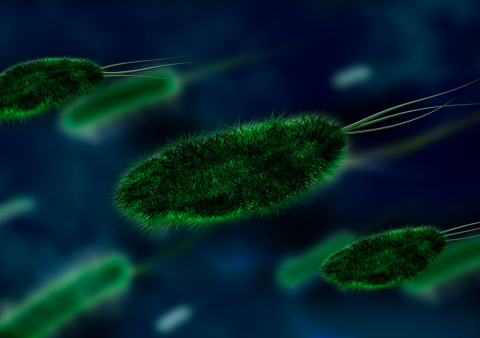
Next Monday is our first edition of internal seminars by PhD students for our QBio/FRESK Seminar. We will welcome Gabriel Mahuas ("How do biological neural networks deal with noise ?") and Tommaso Ocari ("Is my next-generation sequencing deep enough ?").
Abstract:
Gabriel Mahuas
How do biological neural networks deal with noise?
Many biological systems can be seen as information processing ensembles, composed of many interacting agents that receive external inputs from the environment. To understand how these ensembles operate, we need to understand their collective behavior and how it supports the flow of information in the system.
Sensory neurons encode information about incoming stimuli in their collective activity. This population activity is shaped by two main sources of correlations: first, stimuli tend to contain strong spatio-temporal correlations that will in turn correlate neural activity. Second, neurons receive shared noise from upstream cells and interact with each other such that neural noise is correlated across the network.
Using tools from statistical mechanics (Sessak and Monasson 2008, Mahuas et al. 2023), we found a concise analytical picture revealing how correlated noise affects stimulus encoding by using an expansion of mutual information between stimulus and response in terms of first and second order moments of the population activity. Applied to neural data, our approach sheds new light on the noise structure observed in sensory systems and shows that it may be shaped to favor the encoding of certain stimuli.
Tommaso Ocari
Is my next-generation sequencing deep enough?
Next-generation sequencing (NGS) has revolutionized genomics research since the early 2000s. Thanks to this technique, some fields of evolutionary biology such as directed evolution and deep mutational scanning have benefited from the availability of big data, improving the characterization of variants with desired properties. In these cases, NGS is used to screen biological libraries in order to measure the concentrations of different mutants by reading and counting them. Yet, because of several amplification steps in the experimental protocols, like PCR amplification or bacterial growth, NGS datasets suffer from large noise errors that are often neglected. To gain deeper insight in this phenomenon we have developed a statistical model of the entire NGS protocol, incorporating the exponential processes, inspired by Galton-Watson process theory. Our model predicts a simple proportionality law between the variance and the mean of the counts data, that we have validated in both real data and in-silico simulations. Finally as a practical application, this validated noise model can be used to predict which is the optimal NGS depth for a specific biological library.