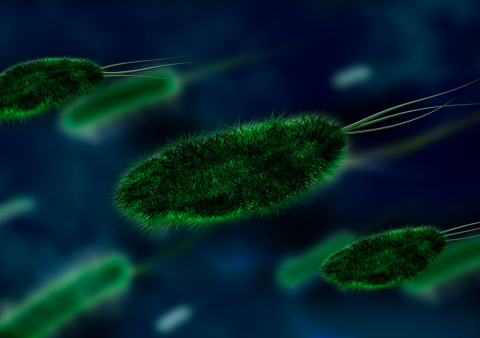
The next FRESK seminar will be hosted by Laurent Jacob (Sorbonne Université) on the theme "neural networks for likelihood-free inference in evolutionary genomics".
Abstract: Statistical inference in evolutionary genomics allows to estimate key parameters such as phylogenetic trees, diversification rates or reproductive numbers of pathogens. This inference relies on probabilistic models that represent how observed homologous sequences evolved given these parameters of interest. Standard approaches such as maximum likelihood or Bayesian inference are well-suited to this problem but require to compute likelihoods, which can become very costly or impossible under realistic (and typically complex) probabilistic models. I will present an alternative likelihood-free approach exploiting samples rather than evaluations of the model. The methods I will present rely on neural network architecture that are specifically tailored to account for the symmetries of each inference problem.