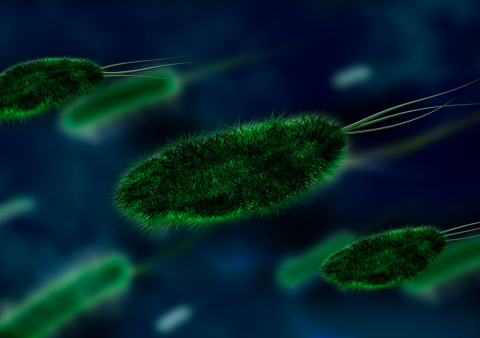
Fil d'Ariane
- Accueil/
- Évènements/
- Séminaire externe - Alessandro Ingrosso (Radboud University, NL)
Pour le prochain séminaire FRESK, Alessandro Ingrosso (Radboud University, NL) donnera une conférence intitulée "Statistical mechanics of transfer learning in the proportional limit"
Abstract :
Learning to generalize from scarce data is a daunting task for biological and artificial neural systems. Transfer learning (TL) has recently emerged as a powerful technique in Machine Learning to boost the generalization performance on a specific (target) task, using information gained from a related (source) task. TL crucially depends on the ability of a neural network to learn useful features. I will present a recent work that leverages analytical progress in the proportional regime of deep learning theory (i.e. the limit where the size of the training set P and the size of the hidden layers N are taken to infinity keeping their ratio P/N finite) to develop a novel statistical mechanics formalism for TL in Bayesian neural networks. We developed an effective theory for TL in one-hidden-layer fully-connected neural networks, using a generalization of the classic Franz-Parisi method to analyze metastable states in spin glasses. I will show how TL is ineffective in the infinite-width limit due to the so-called lazy-training phenomenon. When the number of data points and hidden neurons are comparable, Transfer Learning occurs due to a renormalized source-target kernel that quantifies task relatedness and determines whether TL is beneficial for generalization.